Prof. Jorge Silva from Universidad de Chile visited our group to discuss future collaboration on applications of information theory to predictive geometallurgy.
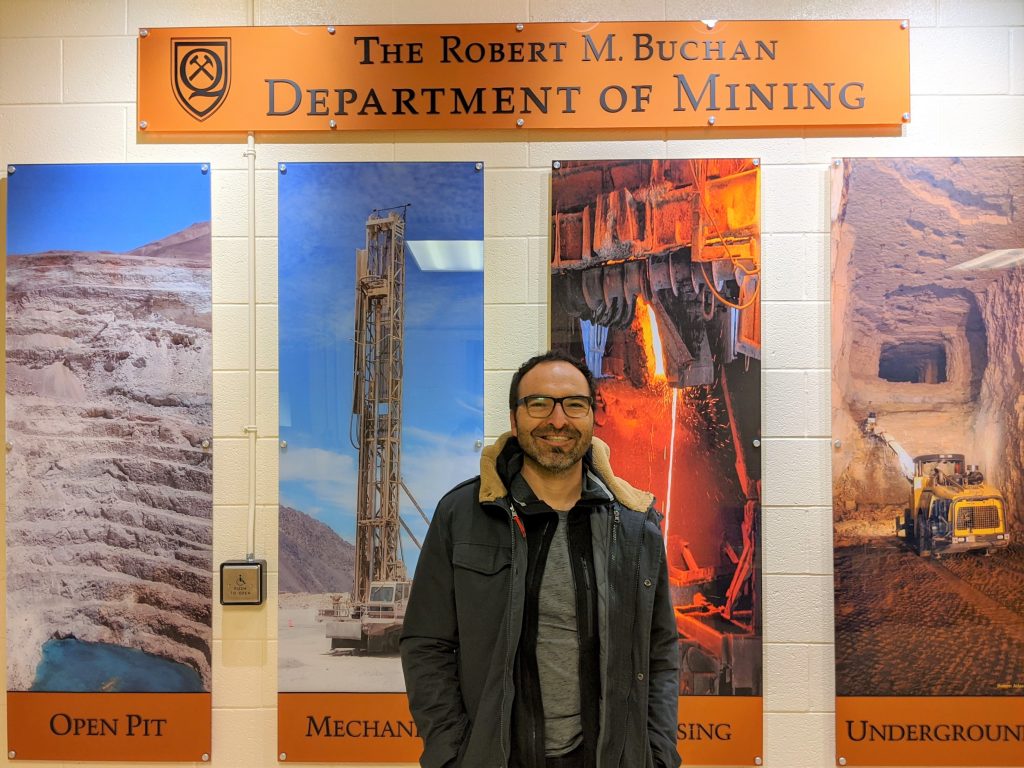
Prof. Jorge Silva from Universidad de Chile visited our group to discuss future collaboration on applications of information theory to predictive geometallurgy.
This paper was recently accepted for publication in Mathematical Geosciences. It presents an approach to reconstruct categorical facies models based on Weighted Compressed Sensing.
The work is part of ongoing collaboration with Prof. Jorge Silva at the Department of Electrical Engineering, at Universidad de Chile.
The paper can be accessed here.
Hernan Calderon (Information and Decision System Group (IDS), Department of Electrical Engineering, Universidad de Chile), Felipe Santibañez (Information and Decision System Group (IDS), Department of Electrical Engineering, Universidad de Chile), Jorge F. Silva (Information and Decision System Group (IDS), Department of Electrical Engineering, Universidad de Chile), Julian M. Ortiz (The Robert M. Buchan Department of Mining, Queen’s University), Alvaro Egaña (Advanced Mining Technology Center, Universidad de Chile)
Abstract A weighted compressed sensing (WCS) algorithm is proposed for the problem of channelized facies reconstruction from pixel-based measurements. This strategy integrates information from: (i) image structure in a transform domain (the discrete cosine transform); and (ii) a statistical model obtained from the use of multiple point simulations (MPS) and a training image. A method is developed to integrate multiple-point statistics within the context of WCS, using for that a collection of weight definitions. In the experimental validation, excellent results are reported showing that the WCS provides good reconstruction for geological facies models even in the range of [0.3–1%] pixel-based measurements. Experiments show that the proposed solution outperforms methods based on pure CS and MPS, when the performance is
measured in terms of signal-to-noise ratio, and similarity perceptual indicators.
The research Annual Report 2019 is now available. Papers can be downloaded and are open to everyone.
This paper was published in a new research journal supported by the Instituto de Ingenieros de Minas de Chile (IIMCh). This is work done by the Master student Rodrigo Gutierrez, while Dr. Ortiz was at Universidad de Chile. The paper can be downloaded here.
R. Gutierrez (Department of Mining Engineering, Universidad de Chile, Avda. Tupper 2069, Santiago 837 0451, Chile), J. M. Ortiz (The Robert M. Buchan Department of Mining, Queen’s University, 25 Union Street, Kingston, ON K7L 3N6, Canada)
ABSTRACT: The definition of mineralized units is key to the predictive capacity of a resource model, since these units define homogeneous volumes where spatial estimation or simulation of the relevant grades can be performed. In this paper, we adapt sequential indicator simulation to model mineralization units in a large porphyry copper deposit, accounting for the weathering profile that defines the vertical zoning of these units. A locally varying anisotropy field is created from the geological interpretation of the contact between the mixed mineralized unit, where the rock mineralization transitions from supergene to hypogene sulphides. A sequential indicator simulation routine is modified to account for the local variations of the units, and all distances are computed through these folded surfaces. Sensitivities related to the main parameters of the simulation algorithm that accounts for the locally varying anisotropy are performed to select the optimum parameters. The final result is compared with conventional sequential indicator simulation, against the geological units logged in blast holes, at a much denser grid, showing an increase in the accuracy in predicting the mineralized unit from the drillhole logged data.
During APCOM 2019, our group presented three papers:
Our research group is having a good time in Montreal. Students are presenting posters and oral presentations of different research topics.
Two papers have been published in collaboration with colleagues from Universidad de Chile and University of Stuttgart in the journal Minerals Engineering.
Studying the integration of solar energy into the operation of a semi-autogenous grinding mill. Part I: Framework, model development and effect of solar irradiance forecasting
Studying the integration of solar energy into the operation of a semi-autogenous grinding mill. Part II: Effect of ore hardness variability, geometallurgical modeling and demand side management
The papers can be donwloaded here (part I) and here (part II).
Sebastian presented his research in the “3 minutes thesis competition” at Queen’s University. Great work!
Ilkay was at PDAC in Toronto, presenting his work in collaboration with Nexa Resources, on the application of Random Forest and PCA to support geological interpretation of geochemical data. This work is done in collaboration with Dr. Gema Olivo from the Department of Geological Sciences and Geological Engineering, at Queen’s University.
You can download the poster below.
New paper in Mathematical Geosciences to start 2019, showcasing the work of Felipe Santibanez, Ph. D. student at Universidad de Chile, in collaboration with Prof. Jorge Silva. You can download it here.
Felipe Santibañez (Information and Decision System Group (IDS), Department of Electrical Engineering, University of Chile), Jorge F. Silva (Information and Decision System Group (IDS), Department of Electrical Engineering, University of Chile), Julian M. Ortiz (The Robert M. Buchan Department of Mining, Queen’s University)
Abstract
The task of optimal sampling for the statistical simulation of a discrete random
field is addressed from the perspective of minimizing the posterior uncertainty of non-sensed positions given the information of the sensed positions. In particular, information theoretic measures are adopted to formalize the problem of optimal sampling design for field characterization, where concepts such as information of the measurements, average posterior uncertainty, and the resolvability of the field are introduced. The use of the entropy and related information measures are justified by connecting the task of simulation with a source coding problem, where it is well known that entropy offers a fundamental performance limit. On the application, a one-dimensional Markov chain model is explored where the statistics of the random object are known, and then the more relevant case of multiple-point simulations of channelized facies fields is studied, adopting in this case a training image to infer the statistics of a nonparametric model. In both contexts, the superiority of information-driven sampling strategies is proved in different settings and conditions, with respect to random or regular sampling.