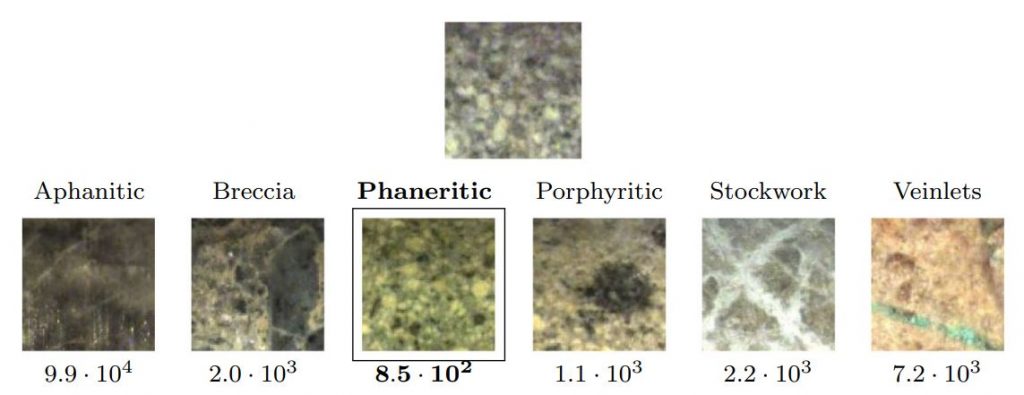
In the May 2020 issue of Mathematical Geosciences, the paper Variogram-based descriptors for comparison and classification of rock texture images was published. This work is the result of the Ph.D. in Mining Engineering of Gonzalo Diaz, at Universidad de Chile, and is the result of collaboration with Prof. Jorge Silva, hist student Rodrigo Lobos, and the researcher from ALGES laboratory, Alvaro Egaña. The paper can be downloaded here.
Variogram-Based Descriptors for Comparison and Classification of Rock Texture Images
Gonzalo F. Diaz (Department of Mining Engineering / Advanced Mining Technology Center, Universidad de Chile), Julian M. Ortiz (The Robert M. Buchan Department of Mining, Queen’s University), Jorge F. Silva (Department of Electrical Engineering, Universidad de Chile), Rodrigo A. Lobos (Department of Electrical Engineering, University of Southern California), Alvaro F. Egaña (Department of Mining Engineering / Advanced Mining Technology Center, Universidad de Chile)
Abstract
Rock characterization is typically performed by geologists in mining companies and involves the analysis of several meters of drill-hole samples to describe distinctive geological properties. In this procedure, rock texture is not typically taken into account despite its importance given its close relation with metallurgical responses and, therefore, all mineral processes. To support the work of geology experts, this research seeks to obtain rock texture information, discriminating it from digital images through image processing and machine learning techniques. For this purpose, a geologist-labeled digital photograph database was used with different rock texture classes (including geological textures and structures) from drill-hole samples. To characterize rock texture, three texture descriptors based on variographic information are proposed, which summarize data contained in the image pixels, focusing on local structural patterns that numerically describe its texture properties. Then, based on a methodology of image texture comparison, which could be extended to classify different types of rock texture classes, a quantification of the system’s performance was obtained. The results showed a high discrimination among common texture classes using compact variogram-based features that outperformed previous methods applied on the same rock texture database.