The paper “On the use of machine learning for mineral resource classification” by Ilkay S. Cevik, Oy Leuangthong, Antoine Cate and Julian M. Ortiz was recently published in Mining, Metallurgy and Exploration, Vol 38, pages 2055-2073. The paper was developed by proposes a methodology to perform mineral resource classification in a consistent and automated manner with a sequence of machine learning methods. This ensures a simple application that can be easily audited and that can be tuned by a Qualified Person, to ensure results are consistent with the uncertainty associated to the resources and the geology.
The methodology combines a repeated application of unsupervised random forest over subsets of blocks in the model, to determine a distance matrix, which is then clustered to discriminate between different classes of blocks. This is then used in a supervised application of random forest to classify the blocks and determine their probability of belonging to each class (measured, indicated and inferred). The method is demonstrated in real deposits, and compared with a Qualified Person classification done with conventional methods.
The paper can be found here: On the Use of Machine Learning for Mineral Resource Classification | SpringerLink
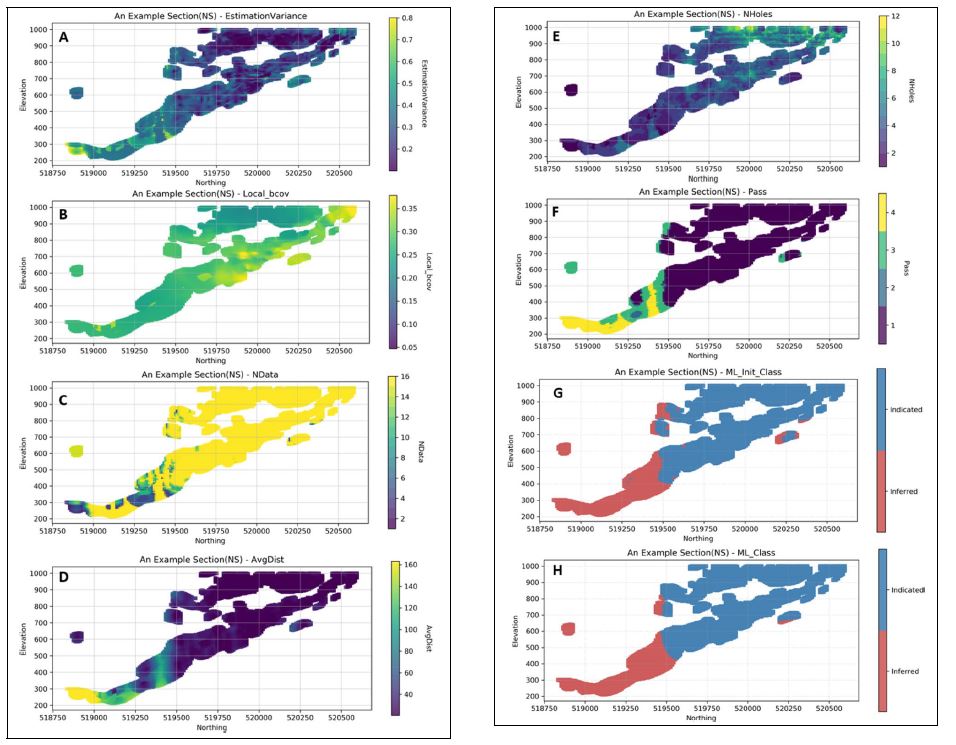